I. Introduction
Informatization promotes convenience and efficiency in our society as an efficient policy tool as well as the driving force behind the development of the country and society. The informatization will solve social problems and create new values for the development of the country and society by the objective analysis of phenomena based on data accumulated so far.
Advanced information technology is essential infrastructure that has moved into society at large, and is becoming the heart of lifestyle and social innovation (Kyung, 2015b). As the modern society develops, risks and uncertainty increase, and information technology and data become means to solve the problem. Particularly, four key information technologies of ICBM (IoT(Internet of Things), cloud, big data, mobile) make the modern society evolve into the human-centered hyper-connected society and the intelligent society, and try to create new values that can resolve risks and uncertainty by means of data generated from them. Among them, big data is emerging from the center as a new paradigm and growth engine of information technology. Big data is technology that can be used as a foundation for the enhancement of industrial competitiveness, the creation of new services and products, and the efficiency enhancement and innovation of public service, through providing support to scientific and rational decision-making, and forecasting the future. Major countries such as the US, EU, Japan, and China are concentrating on the promotion of policies for the vitalization of big data industry in their own countries and the strengthening of its global competitiveness.
It will be possible for big data to be employed usefully in the area of Real Estate due to their close correlation in their characteristics ( Kyung Jung-ik, 2014). That is, big data is one of methods that can solve various problems of Real Estate by collecting and analyzing a large quantity of diverse structured and unstructured data on Real Estate.
To look into previous studies related to this study that intends to vitalize the introduction of big data in the area of Real Estate, they suggest that for the efficient construction and utilization of big data, it is necessary to perceive the strategic value of the introduction of big data, and that it is important to judge whether it is a suitable system for a corporation in terms of an environment for its introduction (Gartner, 2012; Park Jong-man et al., 2012; Yang Chang-joon, 2012; Lee Man-jai, 2012; Sumarker & Ferrell, 2013).
The purpose of this study is to define factors that influence the introduction of big data in the area of Real Estate, on the basis of previous studies that were conducted to derive strategic values and environmental factors for the introduction of the existing information systems or big data. That is, this study intends to suggest guidelines for big data adoption, by clarifying which factors should be focused and promoted in order to introduce big data into the Real Estate section and to use it for the creation of new values and information.
Very few previous studies attempted to derive perception on strategic value and environmental considerations in connection with the introduction of big data in institutions or corporations. Moreover, it may be said that in the area of Real Estate, Kyung Jung-ik (2015) is the only previous study that has relations with the present study. Therefore, this study is going to derive factors having effects on the intention to introduce big data into the area of Real Estate, by investigating factors of successful information system, factors of perception on strategic values, environmental considerations in connection with the introduction of information system, and literature on big data.
First, the spatial target of this study is Korean institutions and corporations concerned with Real Estate. Further, in its contents, it intends to verify the influence that strategic value factors and environmental factors, which are considered at the time of the initial introduction of information system, have on the introduction of big data into the area of Real Estate. That is, it intends to add environmental factors, which failed to be suggested by the existing previous studies, and to verify their influence.
This study reviewed literature on big data and the introduction of information system; and on the basis of the results, derived strategic value perception factors and environmental factors, taking into account the characteristics of Real Estate industry. On this wise, influencing factors were derived and a research model was built, on the basis of previous studies, and detailed measuring variables were developed. To prove the validity of the research model, a questionnaire survey of relevant persons at institutions and corporations where the introduction of big data was anticipated was carried out. The empirical analysis was intended to identify the effects that strategic value perception factors and environmental factors behind the introduction of information system have on the intention to introduce big data into the area of Real Estate.
II. Theoretical Review of the Study
As the speed of technological change and development increasingly becomes faster, the world has been changing more rapidly for recent 20 years than the past 5,000 years or so. Nassim Nicholas Taleb mentions in his work, The Black Swan (2007), that risk is increasing in the modern society beyond our imagination because of the Internet and globalization. Amidst the financial crisis, the real world has seen the phenomena of aggravated social unrest, such as serious depression, conflicts between classes, unstable employment, and deepening polarization.
In other words, in proportion to the width and speed of changes in all the areas of economy, technology, and society, uncertainty increases more, and thus the importance of future forecasting is further increasing.
At this point of time when these phenomena occur, big data is at the heart of development based on information technology in all areas of society. That is, the emergence of big data is inevitable due to environmental changes, given conditions, and necessities, as follows: First, the development of information technology has reached its limits, and the center of its development has been switched from hardware, software, and network to data utilization. In other words, there occur a change in the mind-set to overcome the limits of advancement in information technology through data utilization, and a switch in environment to one that can utilize data in actuality. Second, an environment has been created in which a large quantity of data can be stored and utilized unlike the past, for expenses for network access, hardware manufacture, and data storage have been considerably lowered. Third, owing to data increased by advancement in information technology (IT), the existing techniques for data storage, management, and analysis have reached the limits that can’t be overcome by them; and thus big data that can lead paradigmatic changes to solve the problem has emerged.
It is forecast that the utilization of big data will rapidly spread as the range of data application expands further thanks to progress in the existing concept of data and the development of high-performance computers. And risk factors and complexity increase as the speed of social change and development is accelerated, and thus, the necessity for systematically and promptly sensing and coping with changes in environment increases. Further, there has been increasing necessity for strategic means that can improve insight into the future, coping ability, competitiveness and creative power through the analysis of big data, and can achieve the continuous development of the country. In addition, corporations also expect, from the efficient utilization of big data, cost reduction, advanced decision-making, the prompt grasping of customer propensity, the enhanced accuracy of future forecasting, and the discovery of meaningful patterns.
30% of corporation across the world have already ‘implemented’ and ‘utilized’ big data ( Techpro Research, 2015); and according to a 2014 survey of global corporations conducted by Gartner, 73% responded that they ‘had already invested in big data technology or planned to invest in it within two years.’ An increase in the introduction of big data from 20% in 2013 to 30% in March 2015 supports every organization’s positive forecasting; by industries, it has been found that 58% in the telecommunication sector had experience of a big data project, 45% in the manufacture sector, and 38% in the public sector, respectively (Techpro Research, 2015).
It is forecast that big data will solve complicated optimization problems in every area including transportation, medicine, and industrial automation; realize their potentials; and provide visible benefits in the form of the automation and disruptive innovation of all economic sectors that are based on machine-generated data (IBRS, John Bettin).
In Korea also, for jumping up to the ‘data superpower’ matching its reputation as the ‘ICT infrastructure power,’ the government has promoted the industrial development and active utilization of big data, by establishing big data strategies such as the 2013 December strategy for big data industry development intended to secure global competitiveness and effective support to major government projects such as creative economy and Government 3.0 and the 2014 December strategy for data development. Accordingly, Korea’s big data market is growing at an average annual rate of 27%1) ( National Information Society Agency, 2015).
Every nation’s leaders and corporate managers have changed their perception on big data because of the usefulness of big data as mentioned above, and big data is being perceived as an importance source of competitive advantage and values, beyond the simple perception of it as data used in the existing job performance. That is, a government or a corporation that makes good use of big data can find opportunities for competitiveness and a new growth.
McKinsey presented five social and economic values of big data: increasing industrial efficiency; discovering consumer needs and forecasting trends; providing customized service; supporting optimum decision-making; and innovating business model, products, and services.
Sabherwal & King (1995) said that ‘the strategic applications of information systems’ have serious effects on the survival and growth of corporations by supporting the establishment of corporate strategies or providing a direct or indirect help to the implementation of corporate strategies. Tallon et al. (2000) classified the types of IT strategies to support the two dimensions of business strategy, operational effectiveness and strategic positioning, into the internal dimension and the external dimension. In regard to the purpose of building and utilizing information systems, Jang Gui-suk (2001) argues that the information system is used as a tool for securing a company’s strategic advantage as well as is used to enhance internal business efficiency and reduce cost.
Companies’ investment in IT used to be considered in terms of what good results would be obtained from the development of information systems; recently, however, factors of multiple influences that IT systems have on other areas to which they are applied are being considered as more important. Therefore, the purpose of information system introduction is expanding from the focus on direct profits and cost reduction to corporate strategic value perception and the utilization of IT systems for corporate competitiveness based on the perception. Many researchers including Miles (2009) argue that CEO’s decision on IT investment at a company may depend on strategic value perception on investment in the system (Miles, 2009; Elizabeth et al., 2004; Kim Jin-soo et al., 2011). In a study on the performance of IT investment, Li & Ye (1999) argues that CIO’s and CEO’s perception on strategic values may act as an important factor on the introduction of information system. In addition, Lee (2001) mentions that given the multi-level value model according to a company’s IT utilization, mere the introduction and operation of information system do not achieve value and performance, but performance is created by diverse factors such as strategic values connected with IT.
Recently, based on these many previous studies, investigation is made into factors that have effects on the strategic values of the introduction of information system and big data, such as the aspect of products and services, the aspect of management productivity, and the aspect of decision support. First, in ‘the aspect of products and services,’ the factor of ‘the competitiveness of products and services’ factor and the factor of ‘the capturing of opportunities’ are being considered. ‘The competitiveness of products and services’ is said to have positive effects on the strategic value of information system, for problems can be resolved effectively by means of big data ( Steve et al., 2011; Chau & Xu, 2011; McAfee & Brynjofsson, 2012). In addition, ‘the capturing of opportunities’ refers to grasping new patterns on the basis of data accumulated in daily life and finding customer needs that fail to be recognized by customers themselves or competitors (SERI, 2013).
Next, in ‘the aspect of management productivity,’ ‘the shortening of business hours’ and ‘improvement in business process’ are being considered. McKinsey (2011) mentions that the utilization of big data will lead to shortened business hours, which will attain the effects of improvement in industrial productivity by about 0.5-1.0%. Miles (2008), Kim Eun-jeong (2008), Kim Jin-soo et al. (2010), and Steve et al. (2011) report that the introduction of big data enables the discovery of opportunities for improving business process. And Chau & Xu (2011) mentions that big data makes it possible to monitor major corporate activities, supporting the improvement of corporate business process.
Next, in ‘the aspect of decision-making support,’ corporate capability to systematically analyze diverse data generated and built by information systems inside and outside a company can support an executive’s or a manager’s decision-making effectively ( Quinn & Baily, 1994; Lau et al., 2011). Mckinsey (2011) and Gartner (2011) mention that the analysis of big data enables the monitoring of information inside and outside a company and the analysis of trend changes and risk factors, which can provide information necessary for a corporate manager’s or an executive’s decision-making.
As for the study on influence on the introduction of big data into the area of Real Estate in terms of strategic value, Kyung Jung-ik et al. (2015) is the only one of that kind. They argued that it is desirable that institutions and corporations, which are considering the adoption of big data, should focus, among strategic values, on improving business process, capturing opportunities, providing information for decision-making, and analyzing and forecasting decision-making.
Kuan and Chau (2001), Zhu (2004), Zhu et al. (2003; 2004; 2006), and Zhu & Kraemer (2005) argue that environmental factors are important influencing factors in the introduction of information technology. Tornatzky & Fleischer (1990) mention that environmental factors in introducing information system refer to factors that influence a company from environment inside and outside the company, and that the inside of an industry in which business is carried out, competitors, suppliers, and public and governmental agencies belong to them. In addition, Kuan and Chau (2001), Zhu (2004), Zhu et al. (2003; 2004; 2006), and Zhu & Kraemer (2005) also state that in case of introducing information technology, such environmental factors are important influencing factors in the adoption of information system. It was also verified in many other studies; and thus the theoretical foundation for the fact that environmental factors influence the adoption of information technology has been provided.
Xu et al. (2004) says that the newer a system, the more the informatization environment of an organization acts as an important factor in a company’s introduction of new technology.
In studies on the adoption of EDI, Chwelos et al. (2001) and Kuan & Chau (2001) argue that pressure inside a company and external environment influence the company’s adoption of information system. And Igbaria et al. (1997) argues that a company’s adoption of information system is influenced by organizations outside the company.
To put the above previous studies together, environmental factors that influence the adoption of information system may be classified into the inside of industry, the outside of industry, and public sector. In the course of carrying out business, a corporation is influenced by external industries as well as changes in the environment of its relevant industry. Such changes in environment have effects on the aspect of information technology as well as the corporation’s business area ( Igbaria, M., Zinatelli et al., 1997). And a corporation that experiences severer competition within market comes under stronger pressure to accept information technology; and it may be said that such pressure from the inside of industry is an important factor that influences a company’s IT adoption (Zhu, K., Chau, P., 2001). As for the public sector, it refers to the existing characteristics of an organization. And in general, the organization characteristics include corporation, size, the degree of centralization, formalization, management organization, human resources, and resource slack.
III. The Design of Study
As seen in the previous studies, the range of big data utilization is wide, ranging from the efficiency of internal business performance to the forecasting of external risk and the discovery of opportunities, and thus strategic thinking is important. As shown in the previous studies described above, it is found that strategic values and environmental factors profoundly affect the introduction of big data, compared with the existing information systems ( Mckinsey, 2011; Gartner, 2011; McAfee & Brynjofsson, 2012).
Therefore, on the basis of the perception on the strategic value of information system, and the factors in the successful introduction of big data and information system, this study selected the aspect of products and services considering the characteristics of Real Estate industry, the aspect of management productivity, aspect of decision support, and environmental factors (the inside of industry, the outside of industry, and the public) as independent variables; and the intention to introduce big data into the area of Real Estate as a dependent variable. With these variables, the research model was constructed as shown in Fig. 1.
As shown in the research model, based on strategic values and environmental factors in the introduction of information system, measurement variables and their operational definitions were established in terms of the intention to adopt big data, as follows:
As seen in the previous studies above, many studies suggest as the strategic values of information system adoption various aspects including the aspect of products and services, the aspect of management productivity, and the aspect of decision support (Quinn & Baily, 1994; Miles, 2008; Steve et al., 2011; Chau & Xu, 2011; McKinsey, 2011; Chau & Xu, 2011; Steve et al., 2011; McAfee & Brynjofsson, 2012; SERI, 2013; Kim Eun-jeong, 2008; Kim Jin-soo et al, 2010).
Unlike the existing information systems, big data provides meaningful information by analyzing data that could not be analyzed before. Big data is said to be able to provide services that improve the competitiveness of products and services through personalization and intelligence, by analyzing diverse patterns based on data and forecasting the future, and capturing a sign of danger in advance and enabling real-time decision-making. Big data enhances insight into the future, coping ability, competitiveness, and creativity (National Information Society Agency, 2013). And McKinsey (2011) and Gartner (2012) suggest five major areas of differentiated performance that can be created by big data, that is, the forecasting of the future, the discovery of hidden needs, reduction in risks, customized service, and real-time response. These five major areas of utilization can enhance the management productivity of products and services in the area of Real Estate; and can provide strategic values that can ultimately improve the competitiveness and performance of a company by providing diverse information on effective risk management and decision-making, together with improved ability in analysis and monitoring (Kyung Jung-ik, 2015).
Therefore, by referring to the previous studies on information system and big data, this study selected the products and services, the management productivity, and the decision support as measurement variables, which are factors that influence the introduction of big data into the area of Real Estate; and their operational definitions and related studies are as shown in Table 1.
Variable | Operational definition | Related literature |
---|---|---|
Products and services | The degree of big data’s contributing to the improvement of products and services, sensing of risk, and the discovery of opportunities | Steve et al.(2011) Chau & Xu(2011) McAfee & Brynjofsson(2012) Steve et al.(2011) Quinn & Baily(1994) Miles(2008) Kim Eun-jeong(2008) Mckinsey(2011), Gartner(2012) SERI(2012) Kyung Jeong-ik(2015b) |
Management productivity | The degree of big data’s contributing to the shortened business hours, cost reduction, improved profitability, and improved business | |
Decision support | The degree of big data’s supporting the decision-making of user, manager, and CEO |
Next, to look into the previous studies on environmental factors in the introduction of information system, they are mostly studies on external environment. In a study on EDI adoption, Iacovou et al. (1995) suggested the environmental factor of external pressures as a significant factor.
Mansfield (1997), Zhu & Kraemer (2005), and Zhu et al. (2004, 2006) showed in their empirical studies that the severer the competition in the market, the stronger the pressure to accept information technology. And Jeyaraj et al. (2006), Sharma et al. (2007), and Matta & Moberg (2006) identified external pressure and the degree of competition as important factors that influence the diffusion and promotion of IT adoption.
Besides this competitive environment, governmental regulations were used as an environmental factor. Umanath & Cambell (1994) empirically analyzed that changes in government regulations have significant effects on a company’s adoption of information system; and Dasgupta et al. (1999) and Zhu & Karemer (2006) also showed that government policies have significant effects on companies’ adoption of information system.
Zhu et al. (2006) argued that regulatory environment is an important factor that influences the diffusion of IT innovation, and has effects on changes in companies’ IT environment.
In addition, Mansfield (1997), Sharma et al. (2007), Zhu & Karemer (2006), and Zhu et al. (2006) mention that a corporation facing severer competition comes under stronger pressure to accept information technology, and that such pressure from the inside of industry is a factor that has great effects on a company’s IT adoption.
On the basis of the above previous studies, this study adopted the three measurement variables of ‘the outside of industry,’ ‘the inside of industry,’ and ‘public environment’ as environmental factors that have effects on the intention to adopt big data; and the operational definitions of the variables are as shown in Table 2.
IV. Statistical Analysis
Domestic studies that tried to derive and apply factors having effects on the intention to introduce big data into the area of Real Estate is so few that there is only one study carried out by Kyung Jung-ik (2015). Cases of big data application in domestic Real Estate industry are also insufficient; some are restricted to the level of pilot test, and relevant persons’ level of knowledge about big data is not very high as well. Therefore, this study selected a sample given this situation, and conducted surveys twice from April 22, 2015 to May 6, 2015, and from July 23, 2015 to August 12, 2015, respectively. 165 copies of the questionnaire were collected, among which 152 copies, excluding 13 insincere responses, were subjected to statistical analysis, using SPSS V.23 and Amos V.23.
As for the characteristics of participants in the surveys, those who engaged in Real Estate industry including Real Estate development, sales, brokerage, and appraisal were 56.5%, public officials and public institution staff were 21.1%, and graduate students were 22.4%; and thus it was attempted to collect perceptions on big data adoption from diverse areas of Real Estate.
Variable | Measurement item | Previous researcher | |
---|---|---|---|
Competitiveness of products and services(B) | Can provide customized and intelligent products and services. | B1 | Steve et al.(2011) Chau & Xu(2011) McAfee &Brynjofsson(2012) Chen et al.(2011) McKinsey(2011) Gartner(2011) SERI(2012) |
Helps improve the process for providing a company’s products and services. | B2 | ||
Improves ability to cope with and support customers’ requirements for products and services in real time. | B3 | ||
Improves ability to cope with product or service risk factors in real time. | B4 | ||
Capturing of opportunities(C) | Improves ability to capture opportunities for improvement in business process. | C1 | McAfee &Brynjofsson(2012) Chen et al.(2011) McKinsey(2011) Gartner(2011) SERI(2012) |
Improves ability to capture opportunities for the improvement of products and services. | C2 | ||
Improves ability to capture opportunities for the development of a new product and service. | C3 | ||
Improves ability to capture opportunities for business convergence and a new business. | C4 | ||
Shortening of business hours(D) | Can shorten the time of product and service production/distribution. | D1 | Elizabeth &Michael(2004) McKinsey(2011) Gartner(2011) Steve et al.(2011) OECD(2011) Kim & Ka(2012) Kim Y. M.(2013) |
Can shorten the time to cope with a customer’s claim & requirement. | D2 | ||
Can shorten the time to analyze and predict changes in corporate internal (products/services, production and distribution) and external (customer, competitor, market) environment. | D3 | ||
Can shorten the time of executive’s and manager’s decision-making. | D4 | ||
Can shorten the period of new product and service development. | D5 | ||
Can shorten the period of new business model development and convergence with other area. | D6 | ||
Improvement in profitability(E) | Can increase sales. | E1 | Elizabeth &Michael(2004) McKinsey(2011) Gartner(2011) Steve et al.(2011) OECD(2011) Kim & Ka(2012) Kim Y. M.(2013) |
Can increase market share. | E2 | ||
Can improve customer satisfaction. | E3 | ||
Can improve ROI (return on investment). | E4 | ||
Improvement in process(F) | Can improve the process for product production (distribution) and service providing. | F1 | |
Can improve the process for analyzing and forecasting the status inside and outside company. | F2 | ||
Can improve the process for executive’s and manager’s decision-making. | F3 | ||
Can improve the process for the development of new product and service. | F4 | ||
Can improve the process for the development of new project and business. | F5 | ||
Decision support(G) | Can effectively support decision-making for coping with issues and risks. | G1 | Quinn & Baily(1994) McKinsey(2011) Gartner(2011) Steve et al.(2011) Kim & Ka(2012) |
Can provide diverse information for decision-making, based on social phenomena and actual data. | G2 | ||
Capable of diverse analyses (social analysis, credit analysis, reputation analysis) and prediction for decision-making | G3 | ||
Outside industry(H) | Degree of coping with changes in IT trends and IT environment | H1 | Iacovou et al.(1995) Mansfield(1997) Zhu & Kraemer(2005) Zhu et al.(2004, 2006) Umanath & Cambell(1994) Sharma et al.(2007) Dasgupta et al.(1999) |
Degree of coping with changes in IT environment in other industries | H2 | ||
Degree of big data adoption in companies of other areas | H3 | ||
Inside industry(I) | Degree of coping with changes in IT trends and IT environment within industry | I1 | |
Degree of big data adoption in major competitors | I2 | ||
Degree of core business partner’s request and recommendation | I3 | ||
Public environ-ment(K) | Degree of changes in government and public agencies’ policies | K1 | |
Degree of changes in relevant laws & regulations and institutions | K2 | ||
Degree of the increase in big data adoption in the public sector | K3 |
This study selected variables and detailed measurement items whose content validity was confirmed, considering the characteristics of Estate industry2) and theories and previous studies that ensure content validity as to the environmental factors and the strategic values of big data and information system adoption. The selected items were measured with a multiple-item Likert scale.
Variable | Measurement item | Previous researcher | |
---|---|---|---|
Intention to adopt big data in Real Estate area (M) | Considering big data adoption | M1 | Bakos & Treacy(1986) Johnston & Carrico(1988) Elizabeth & Michael(2004) Sharma et al.(2007) |
Has established a human resources plan (education, training, recruitment) for the adoption and utilization of big data | M2 | ||
Has established an informatization plan for big data adoption | M3 |
First, to test the reliability of survey results, reliability was measured by using Cronbach’s α; and to judge the goodness- of-fit of the research model, the goodness- of-fit of the research unit and the research model was evaluated by applying χ2, RMR, GFI, CFI, NFI, IFI, and RMSEA, indicators of goodness-of-fit provided by the structural equation model.
In addition, a structural equation model was used to investigate the relations of effects that the strategic value factors, that is, the products and services, the management productivity, and the decision support, and the environmental factors have on the intention to introduce big data into the area of Real Estate.
First, principal component analysis was conducted with the measurement variables selected from the previous studies, in order to select constructs through scale purification process; and a varimax rotation was adopted to simplify factor loadings. Criteria for selecting acceptable measurement items in this study were the eigen value of 1.0 or above and the factor loading of 0.4 or above. As a result of conducting factor analysis on independent variables, the same 9 factors as those selected from the review of previous studies were selected; 8 measurement items, which were loaded not suitably to the theoretical structure, were removed; and thus a total of 27 items were finally adopted. As for the dependent variable, 3 items for one factor were adopted. And as a result of evaluating the reliability of measurement items, based on Cronbach α, and examining construct validity, it was found that Cronbach α shows high reliability of 0.746 or above, as shown in Table 6 and Table 7.
In addition, as for the discriminant validity, if a variance extracted between two factors is greater than the correlation coefficient (coefficient of determination, r2) of each factor, it is judged that the discriminant validity has been secured; in this study, it was found that the absolute value of the correlation coefficients of almost all the factors are smaller than 0.8, and thus it is judged that measurement items used in this study secured the discriminant validity.
The results of testing the goodness-of-fit of the model by combining factors whose unidimensionality was confirmed on the basis of confirmatory factor analysis (CFA) show no satisfactory level of goodness-of-fit.
In addition, as for the unstandardized factor loadings and the squared multiple correlations (SMC) between the measurement items and concepts, all parameter estimates about the relationships between the latent variables and the measurement variables show values greater than 0; and all CR values for the estimates also exceed 2, and are good. And SMC show values of 0.4 or higher, and thus it may be said that the latent variables well explain the variance of their relevant measurement variables. In addition, it is judged that the level of reliability for all the variables is good, with their Cronbach’s α being between 0.746 and 0.921.
However, it was attempted to improve the goodness-of-fit of the measurement model by removing additional items from the suggested model twice on the basis of SMC values, as shown in Table 9, or using MI (modification index).
As for criteria for the indices of goodness-of-fit to adopt an optimized model, they were set to 0.9 and above for GFI, AGFI, CFI, and IFI, 0.05 and below for RMR, and 0.1 and below for RMSEA, respectively (Marsh, H. W., Balla, J. R., & Hau, K. T, 1996). The present measurement model shows GFI and AGFI of 0.9 and below; however, given that CFI (comparative fit index), which is free from sample characteristics, is recommended because AGFI can be influenced by inconsistencies caused by sample characteristics, CFI & IFI and RMSEA in this study are found to be 0.9 and above and 0, respectively, which are judged to be on acceptable levels.
Results of verifying the factors, which influence the successful promotion of Real Estate informatization policies, by means of a structural equation model are as follows:
As a result of verification based on the modified model, it was found that, in terms of the strategic value of big data, the capturing of opportunities, the improvement in business process, and the decision support influence the intention to adopt big data in the area of Real Estate. Also, it was found that environment inside Real Estate industry among the environmental factors influences the intention to adopt big data.
This study aimed to empirically analyze and identify factors affecting the intention to adopt big data in the area of Real Estate, on the basis of previous studies on the perception of strategic values and environmental factors.
As a result of testing with the structural equation model, the capturing of opportunities, the improvement in business process, and the decision support among the strategic value factors were extracted as factors that influence the introduction of big data into the area of Real Estate. In addition, among the environmental factors, the factor inside industry was identified as an influencing factor.
That is, the adoption of big data in the area of Real Estate makes it possible to capture opportunities, enhance the efficiency of business performance by improving business process, and provide support to decision -making. Policy reports and research reports by domestic and foreign institutions and corporations (Mckinsey, 2011; Gartner, 2011; SERI, 2013; NIA, 2012; National Information Society Agency, 2011) are evaluating big data as information system that has high value in various areas such as enterprise, government, and public institution. Further, major governments around the world and global enterprises recognize big data as information technology whose strategic utilization will enable a country and an enterprise to strengthen their competitiveness in the future.
It may be said that the findings of this study regarding the strategic values are similar to those of Kyung Jung-ik’s (2015) previous study. That is, it is confirmed again that the capturing of opportunities, the improvement in business process, and the decision support are factors affecting judgment on the strategic value of big data adoption in Real Estate.
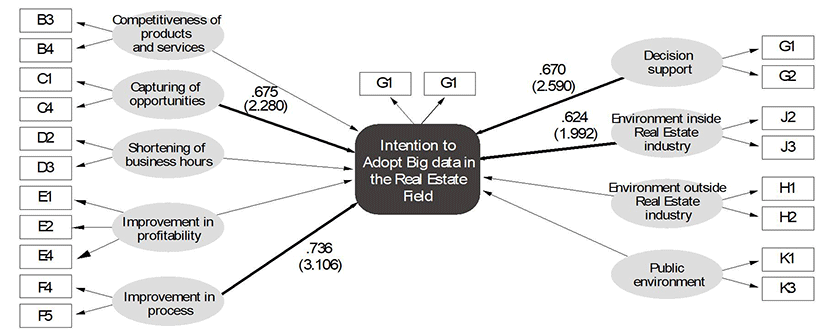
V. Conclusion
Real exchanges influences with diverse areas such as economy, society, politics, culture, and law; and the consciousness and psychology of the majority of people regarding Real Estate have increasingly more effects. It is not easy to consider such diverse factors comprehensively (Kyung Jung-ik, 2013). Real Estate activities involve diverse aspects including Real Estate sale and purchase, lease, brokerage, utilization, development, location selection, policy-making, and pricing; and ultimate analysis and judgment about them are carried out revolving around the three major aspects of Real Estate (technological, economic, and legal), by sing diverse analysis tools. Big data will enable predictable Real Estate activities by resolving such complex and complicated problems of Estate (Kyung Jung-ik, 2014). This study intended to extract factors that affect the intention to introduce big data into the area of Real Estate, on the basis of the perception of strategic values and the environmental factors suggested by Paret et al. (1988), Quinn & Baily (1994), Elizabeth & Michael (2004), and Kyung Jung-ik (2015); and to suggest guidelines to institutions and corporations that review and promote the adoption of big data.
The results of the empirical analysis of this study suggest that institutions and corporations, which consider the adoption of big data in the Real Estate field, should examine the following aspects of strategic values and environmental factors.
First, it should be possible to enhance business efficiency in Real Estate activities (policy, development, investment, etc.) requiring comprehensive consideration about diverse areas including politics, economy, culture, law, etc., by improving the process of business performance.
Second, it will be possible to create new values from converging diverse data on Real Estate including unstructured data such as social data and web log data as well as a variety of structured data on Real Estate. Therefore, in case of adopting big data, the possibility should be considered for capturing new opportunities from the convergence of diverse data on Real Estate.
Third, it will be possible to make an optimum decision, using valuable information provided by big data. It may be said that Real Estate information activities are continuous activities to reduce uncertainty commonly inherent in Real Estate. The adoption of big data should make it possible to minimize uncertainty by collecting and analyzing diverse unstructured data on Real Estate, which could not be utilized before, and to make a decision based on real-time analysis and forecasting.
Fourth, big data will enable prediction with patterns and trends derived from the past and present diverse data. Prediction based on big data is an important factor that should be considered in case of making a decision on Real Estate activities (Kyung Jung-ik, 2015).
Fifth, the factor of environment inside Real Estate industry will have to be reviewed fully in case of introducing big data into the area of Real Estate.
This study is a follow-up study in which environmental factors are added to the factors of strategic values in Kyung Jung-ik (2015); and aimed to extract factors that influence the intention to adopt big data in the area of Real Estate.
Studies on the adoption of big data in the area of Real Estate are still in their early stages, and the following will have to be complemented in future studies.
First, this study surveyed specialists and graduate students in the area of Real Estate; some subjects, however, failed to understand the contents of the questionnaire, and thus there were limitations in the survey on the perceptions. Therefore, the selection of subjects needs to be carried out carefully, and it is necessary to explain about big data fully before survey.
Second, this study has limitations in that it was conducted on the basis of previous studies on the strategic values of information system and environmental factors, as there are insufficient previous studies on the adoption of big data in the area of Real Estate. There will have to be in-depth studies of influences on the intention to introduce big data in the area of Real Estate, through further expansion of studies on the adoption of big data in the area of Real Estate and sufficient discussions.
Third, this study investigated the whole Real Estate industry, without considering characteristics by Real Estate field, and thus it has limitations in practical application. Therefore, future studies will need to raise actual applicability by considering the characteristics of Real Estate evaluation, policy, consulting, brokerage, etc.